Blog Post < Previous | Next >
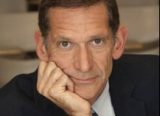
Michael Barnes
The Naive Economics of SB 50: Part I - Understanding Supply and Demand
San Francisco State Senator Scott Wiener, along with our own State Senator Nancy Skinner (in the news recently), have resubmitted their zoning bill SB 50, which was converted to a two-year bill at end of the last legislative session. The final version of the bill is not yet available, but the flaws in previous incarnations of this bill no doubt will remain.
The rhetoric from the bill’s supporters has been sloppy enough that I think it’s time to frame the issues the bill raises in the rigorous analytic framework of neoclassical economics. SB 50’s emphasis on housing supply recalls the supply-side economics of the Reagan administration. But neither supply-side economics nor SB 50 are based on mainstream economics.
In what follows, I’ll lay out the groundwork my analysis, which will be familiar to any undergraduate economics major. I know this because in the early 1990s, I taught economics at UC Berkeley as a graduate student instructor and as an acting instructor.
Here’s my first question, which is one that could have been drawn from a quiz early in an intro econ course: In a market with a standard downward sloping demand curve and upward sloping supply curve, in order to lower equilibrium price and raise equilibrium quantity, is it sufficient to shift the supply curve outward? If not, what other conditions must be assumed?
Figure 1: SB-50’s implicit vision of the housing market.
Click on the images in this article to enlarge
Figure 1 describes, in a standard intro econ graph, the question posed. In a housing market, assume a fixed demand curve (D), and an outward shift in supply from S1 to S2. The price of housing falls from P1 to P2, while the quantity of housing supplied rises from Q1 to Q2.
This is a result that proponents of SB 50 like to assume. But the result rests upon a very strong, and very unrealistic assumption–that the demand curve for housing is fixed. In the Bay Area, the demand for housing has shifted outward at a dramatic rate, driven by the growth of large monopolistic tech firms like Apple, Google and Facebook, and by the billions of dollars of venture capital being funneled to tech startups here. This growth requires more tech workers, more office buildings and ultimately more housing.
The choice of the expression “housing crisis” was a deliberate, misleading attempt on the part of SB 50 supporters and other pro-growth advocates to shape the debate. The state’s Office of Housing and Community Development (HDC) instead uses the term “housing shortage.” Statewide, the shortage is the result of both a physical shortage of housing and an income mismatch that HCD estimates requires the construction of 1.5 million units of affordable housing for the poorest of California’s residents.
If simple poverty is the major problem for the whole state of California, in the Bay Area the problem is mostly due to relative poverty–the influx of a highly paid cohort of tech workers crowding out lower-income residents. This is not a housing crisis–there was no hurricane or outbreak of mutant termites that destroyed thousands of apartments. What we have is a venture-capital driven influx of tech workers. It would be more accurate to call this a “housing demand shock.”
Figure 2: In the real world, demand for housing is shifting outward.
Here’s how we could graph a housing demand shock. For simplicity, in Figure 2 above, the supply curve of housing is held constant, while the housing demand shock shifts the demand curve from D1 to D2. While the assumption that the supply curve is constant is too simple, it is very realistic to assume that the demand for housing in California, and especially in the Bay Area, has been shifting outward. In this example, like the example in Figure 1, the equilibrium quantity of housing supplied rises from Q1 to Q2. However, unlike in Figure 1, the equilibrium price rises from P1 to P2.
The outward shifts in supply and demand both cause quantities to rise, while these outward shifts have contradictory effects on prices. To explore this more fully, let’s combine shifts in demand and supply together in one graph.
Figure 3: Demand and supply both shift outward, but demand shifts out more.
In this example, demand and supply curves both shift outward. Equilibrium quantities rise as before, and prices rise somewhat. The price response is moderated by the relatively small outward shift of the supply curve.
When both supply and demand curves are shifting out, it is the relative size of the shifts that matter. By now the reader can probably see that if the S2 supply curve continued to shift out far enough, with its intersection point moving down the D2 demand curve, the new price would be lower, not higher. The reader is encouraged to draw diagrams of their own, not only shifting the positions of the curves, but also drawing them steeper (more price-inelastic) or flatter (more price-elastic).
Three general points should be made here:
1) It is unreasonable to assume that housing supply can shift rapidly enough to accommodate a housing demand shock driven by volatile capital flows. This is especially true because builders of new affordable housing shared in very little of this largess.
2) It is generally faster to build office buildings than new communities. With respect to communities, office buildings require far less services like police and fire departments, schools, parks and libraries and utilities.
3) When they ignore the demand issues, SB 50 proponents violate one of the fundamental concepts of economics–that in a market, prices and quantities are set by the interaction of supply and demand, and not by supply conditions alone.
Linking housing demand and supply
At least in San Francisco, SB 50 isn’t the only game in town. An initiative that explicitly links housing demand and supply will be on the ballot in March 2020. Sponsored by the community development organization Todco, Measure E will cap office construction unless the city meets its affordable housing goals (Links here and here).
Figure 4: Converting office space to housing, especially affordable housing.
While capping office construction to allow new housing to catch up is an idea worth supporting, restoring the jobs/housing balance could still take years–and without something like the Todco proposal it may never happen at all, since SB 50 makes no attempt to control housing demand.
An intriguing possibility to speed up that process is suggested by Figure 4. What if we could increase housing supply and simultaneously decrease housing demand by converting offices to apartments? In such a scenario, small office buildings could be completely retrofitted and converted to apartments, while whole floors of taller office buildings could be converted.
The advantages to this plan are many. Since building any new housing is very expensive, money would be saved by utilizing existing buildings. Downtown office workers could walk to work (or perhaps just take an elevator), and their presence in the neighborhood in the evenings would create a lively after-work social scene with new bars, restaurants and shops. If some of the new apartment units were affordable, inclusionary and affordable housing programs could be tapped for revenue to subsidize the retrofitting.
Of course, this would mean that some tech businesses would leave the city, a trend that is already beginning. But is this so bad? By moving to cities with lower housing costs, tech workers could afford houses instead of apartments, and move into neighborhoods with good schools and other amenities. And they might not have to move far. Oakland, Concord and Walnut Creek, Sacramento, Las Vegas, Phoenix and Austin all are possibilities. It makes sense to move jobs to where housing is more available.
In the story told in Figure 4, as offices start to close and workers move to other cities, the demand curve shift inward from D1 to D2. Housing quantities and prices both fall temporarily. But as the former office spaces are converted to housing, and the supply curve shifts from S1 to S2, housing prices continue to fall while musicians, artists, people of color, students, new immigrants and commuters are drawn back to the city.
This is a story of the de-gentrification of San Francisco. So, to answers the conundrums of balancing supply and demand requires more analysis.
Zoning can be a non-binding constraint
If SB 50’s advocates fail, in part, because they do not grasp the interaction of supply and demand, there remains a deeper failure. Upzoning will not effectively increase housing supply, at least not for many decades, if at all.
Zoning is a constraint, although a complicated one. But in the current housing market in the Bay Area, zoning is mostly a non-binding constraint. That is why changing zoning laws will not be effective in the short run. By definition a “crisis” is something happening here and now and requires effective solutions in the short run. Zoning changes are not that solution.
To discuss this, I want to introduce the topic of constrained optimization in a form that is familiar–the model of consumer behavior in standard neoclassical economics.
Figure 5: A simple model of consumer choice in the present of a budget constraint.
Figure 5 presents a typical graph that could be found in many intro econ textbooks. A consumer is faced with choosing how many mangoes and avocados to purchase. The goal is to maximize utility subject to a budget constraint. To keep things simple, let’s just assume the consumer budgets six dollars per week on fruit, and they only like to eat mangoes and avocados. Mangos cost $1 each and avocados cost $2 each.
If they spend their entire fruit budget of $6 per week, the consumer could buy six mangoes or three avocados, or some combination of the two. Their purchase decision will lie along the blue budget constraint line.
The graph also features a series of indifference curves which display the consumer’s preferences between mangoes and avocados. Along each curve, the consumer is equally satisfied with the options available. As we move to indifference curves further up and to the right, the consumer’s satisfaction, or utility, goes up. That’s another way of stating that more is better, a basic the assumption in these models. The consumer maximizes their utility by reaching the highest indifference curve possible without violating their budget constraint. In this example, our consumer will choose to buy two avocados and two mangoes.
Now let’s assume that due to the popularity of avocado toast, the local grocery where our consumer buys fruit limits customers to one avocado per day, or seven per week. We represent this new constraint on the graph as a dashed vertical blue line at the number seven on the horizontal avocado axis. Now the figure contains two constraints, one binding, and one non-binding.
In this example, the budget constraint is the binding constraint, and the store’s limit is non-binding. But if our consumer was shopping for a family, their budget for fruit might be far larger and their budget constraint could lie much farther to the right. If, for example, our family shopper had a fruit budget of $60 per week and wanted to purchase 20 mangoes and 20 avocados, their choices would be constrained by the grocery store’s limits. In that case, the budget constraint would be non-binding, and the store’s limit would be binding.
Here is another example in a different context: Assume you are a very fast center fielder playing for a major league baseball team. The center field wall is short and you a facing a team with lots of power hitters. For you the outfield wall will be a constraint if the opposing team’s hitters are whacking balls into the grandstands. On the other hand, if you are playing a team that focuses on line drives and high batting averages, the outfield wall may not be a constraint because balls aren’t being hit that far.
The important point to remember is that removing non-binding constraints does not change the equilibrium outcome. Whether or not a constraint is binding or non-binding depends on the situation. In large, complex, multi-dimensional models, it is often not obvious which constraints are binding, and computer algorithms are used to determine the optimum outcome.
So, how does all this work in the real world?
Michael Barnes is a science editor and writer at UC Berkeley and is currently serving as a City Council member in Albany, CA.